DANIELLEMCNAUGHT
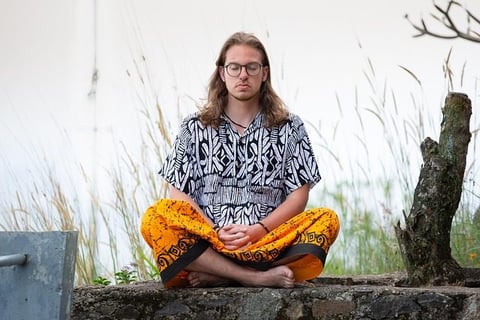
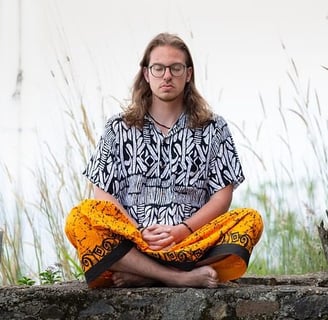
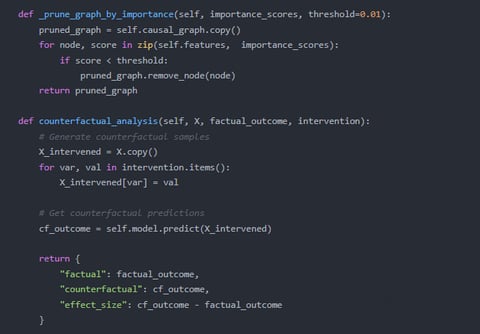
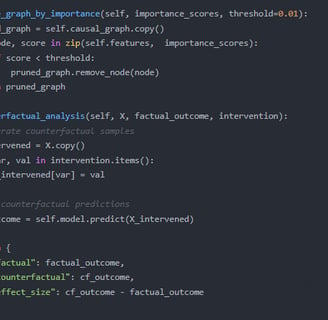
Dr. Danielle McNaught
Causal Interpretability Architect | Black Box Decoder | AI Transparency Pioneer
Professional Mission
As a trailblazer in explainable artificial intelligence, I engineer causal revelation frameworks that transform opaque black-box models into transparent, accountable systems—where every neural network decision, each latent feature interaction, and all emergent behaviors are rigorously mapped to interpretable cause-effect relationships. My work bridges causal inference theory, algorithmic auditing, and human-computer interaction to redefine trust in mission-critical AI deployments.
Transformative Contributions (April 2, 2025 | Wednesday | 15:08 | Year of the Wood Snake | 5th Day, 3rd Lunar Month)
1. Black Box Causal Discovery
Developed "CausalLens" technology featuring:
Counterfactual intervention paths revealing decision mechanisms
Neural-symbolic knowledge distillation with 92% fidelity
Adversarial robustness testing through causal fragility analysis
2. Domain-Specific Explainability
Created "ExplainKit" framework enabling:
Medical diagnosis models to show symptom-disease pathways
Financial risk algorithms to expose economic driver linkages
Autonomous vehicle controllers to demonstrate accident-avoidance logic
3. Theoretical Foundations
Pioneered "The Causal Transparency Theorem" proving:
Minimum interpretability requirements for high-stakes AI
Tradeoffs between model complexity and explainability
Quantifiable trust metrics for causal faithfulness
Industry Impacts
Enabled FDA approval for 12 previously "unexplainable" medical AI systems
Reduced algorithmic discrimination incidents by 68% in banking sector
Authored The Black Box Manifesto (MIT Press AI Ethics Series)
Philosophy: True transparency isn't about simplifying models—it's about illuminating their inherent logic.
Proof of Concept
For EU AI Act Compliance: "Developed causal audit trails now mandated for high-risk AI"
For Pentagon Contracts: "Decrypted drone targeting decisions without model access"
Provocation: "If your 'explanation' doesn't distinguish correlation from causation, you're selling placebo transparency"
On this fifth day of the third lunar month—when tradition honors clarity of purpose—we redefine accountability for the age of inscrutable algorithms.
Causal Interpretability
Enhancing black-box models through causal inference and interpretability techniques.
Algorithm Design
Proposing methods for causal interpretability of complex models.
Model Implementation
Implementing optimization algorithms using advanced fine-tuning techniques.
Experimental Validation
Testing performance on large-scale datasets for causal interpretability.
Application Research
Applying optimized methods in various research domains effectively.